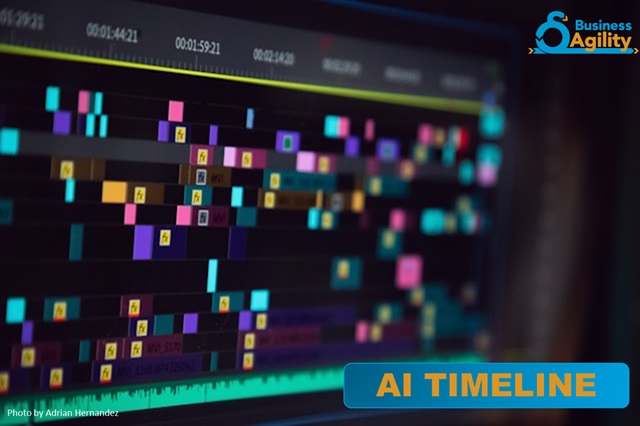
ABSTRACT : L'évolution de l'IA a commencé dans les années 1950 avec des travaux fondamentaux sur le raisonnement symbolique. Au fil des décennies, elle s'est étendue aux systèmes experts, puis s'est orientée vers l'apprentissage automatique et les réseaux neuronaux dans les années 1990. Les percées dans le domaine de l'apprentissage profond dans les années 2000 et la révolution de l'apprentissage profond dans les années 2010, en particulier avec l'avènement des modèles transformateurs, ont marqué des étapes importantes. Les années 2020 sont consacrées à l'amélioration du NLP et à l'intégration de systèmes multimodaux, ouvrant la voie à de futurs développements vers l'AGI. La chronologie prévoit des avancées en matière d'empathie de la machine, de compréhension du contexte et d'intégration de l'IA spécialisée, pour aboutir à l'AGI et à ses profondes répercussions sociétales dans les années 2060 (mais probablement beaucoup plus tôt).
L'évolution de l'IA depuis 1950 et les spéculations sur 2060
Retracer l'évolution de l'intelligence artificielle (IA) depuis ses fondements dans les années 1950 jusqu'à l'avenir spéculatif de l'intelligence générale artificielle (AGI) et au-delà offre une vue d'ensemble de la manière dont ce domaine s'est développé et de la direction qu'il pourrait prendre. Cette chronologie intègre les étapes importantes, les avancées technologiques et le chemin prévu pour atteindre des capacités d'IA plus avancées.
Années 1950-1960 : L'aube de l'IA
- Fondation et optimisme: Le terme "intelligence artificielle" est inventé lors de la conférence de Dartmouth (1956), marquant le début officiel de l'IA en tant que domaine. Les premiers travaux se concentrent sur le raisonnement symbolique et la résolution de problèmes.
- Faits marquants: Développement des premiers programmes d'IA, notamment le programme de jeu de dames d'Arthur Samuel et le Logic Theorist de Newell, Shaw et Simon.
Années 1970-1980 : Expansion et diversification
- Systèmes basés sur des règles: La recherche en IA s'étend aux systèmes experts, qui utilisent des approches basées sur des règles pour imiter le processus de prise de décision des experts humains.
- Les premiers défis: Le domaine de l'IA connaît son premier "hiver", une période de réduction du financement et de l'intérêt, en raison des limites des premières techniques en matière de mise à l'échelle et de traitement de l'incertitude.
1990s : L'essor de l'apprentissage automatique
- Passage à l'apprentissage automatique: L'accent est mis sur le développement d'algorithmes capables d'apprendre à partir de données, ce qui entraîne une résurgence de la recherche et de l'intérêt pour l'IA.
- Les réseaux neuronaux gagnent en popularité: Malgré un deuxième hiver de l'IA, les travaux fondamentaux sur les réseaux neuronaux ouvrent la voie à de futures percées dans le domaine de l'apprentissage profond.
2000s : Percées dans le domaine de l'apprentissage profond
- Progrès dans les réseaux neuronaux: Des avancées majeures, notamment le développement de techniques d'apprentissage efficaces pour les réseaux neuronaux profonds, conduisent à des améliorations significatives dans des tâches telles que la reconnaissance d'images et de la parole.
- Renouvellement de l'intérêt et de l'investissement: La recherche sur l'IA bénéficie d'un financement accru et les entreprises technologiques commencent à investir massivement dans l'IA.
2010s : L'IA se généralise
- La révolution de l'apprentissage profond: Des technologies telles que les réseaux neuronaux convolutifs (CNN) et les réseaux neuronaux récurrents (RNN) sont à l'origine de progrès dans les domaines de la vision artificielle et du traitement du langage naturel.
- Principales étapes: Les systèmes d'IA atteignent des performances comparables à celles des humains en matière de reconnaissance d'images, de reconnaissance vocale et de jeux stratégiques tels que le jeu de Go.
2017 : L'ère des Transformers commence
- Introduction du modèle de transformateur: Le document "L'attention est tout ce dont vous avez besoin" révolutionne la PNL en établissant de nouvelles normes de performance et d'efficacité.
2020s : Spécialisation et intégration
- Progrès continus dans le domaine de la PNL: Développement et perfectionnement de modèles basés sur des transformateurs tels que GPT et BERT, améliorant la compréhension du langage et les capacités de génération.
- Systèmes d'IA multimodaux: Des avancées significatives dans l'intégration de différentes formes de données (texte, images, voix) pour améliorer la compréhension contextuelle de l'IA.
2030s : Théorie de l'esprit et empathie des machines
- Premiers modèles de la théorie de l'esprit: L'IA commence à démontrer des capacités de base pour comprendre les intentions et les émotions humaines dans des contextes spécifiques.
- Développement d'une IA empathique: Des systèmes d'IA capables de reconnaître les émotions humaines et d'y répondre sont mis au point et trouvent des applications dans le service à la clientèle et les soins de santé.
2040s : Compréhension contextuelle avancée
- IA contextuelle sophistiquée: L'IA permet de mieux comprendre des contextes complexes, en intégrant des données multisensorielles et des connaissances historiques.
2050s : La voie vers l'AGI
- Intégration de systèmes d'IA spécialisés: Efforts visant à combiner des IA spécialisées en systèmes cohésifs capables d'apprendre et de raisonner à des fins générales.
- Des prototypes de systèmes AGI voient le jour: Prototypes capables d'effectuer de manière autonome un large éventail de tâches à un niveau humain ou proche de celui-ci.
Les années 2060 et au-delà : Réalisation de l'AGI
- L'AGI devient une réalité: Les systèmes AGI capables d'innovation et de créativité transdisciplinaires transforment la recherche scientifique, l'éducation et l'industrie.
- Transformation de la société: L'impact de l'AGI entraîne des changements majeurs dans le fonctionnement des sociétés, notamment dans l'économie, l'éducation et l'éthique.
Considérations et défis
- Implications éthiques et sociétales: La voie vers l'AGI sera marquée par des débats éthiques et la nécessité d'une gouvernance solide pour garantir que les avantages de l'IA soient équitablement répartis.
- Incertitudes technologiques: Les prévisions sont susceptibles d'être modifiées en raison de percées potentielles, d'obstacles technologiques et de la nature dynamique de la recherche sur l'IA.
Cette chronologie retrace l'évolution de l'IA depuis ses débuts théoriques jusqu'à son état actuel et se projette dans un avenir où l'IA et au-delà pourraient modifier radicalement notre relation avec la technologie et les autres.
Photo par Adrian Hernandez